Introduction
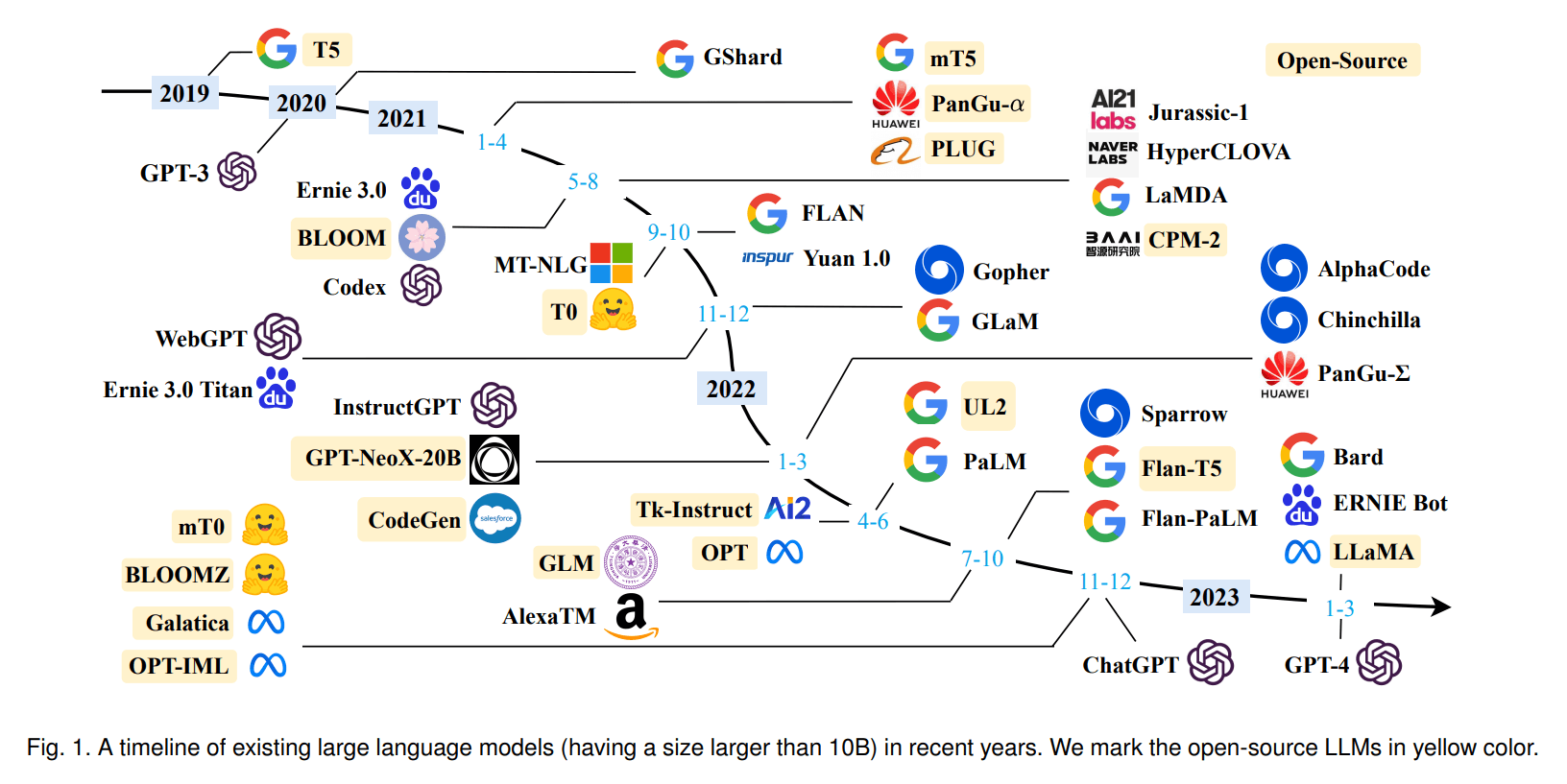
Background
The roots of text generation can be traced back to early AI research in the late 20th century. Initial approaches were rule-based, relying on predefined templates to create simple sentences or paragraphs. However, these methods were limited in flexibility and realism. The evolution of machine learning, particularly with the advent of deep learning techniques, has drastically altered how text generation systems operate.
In the early 2010s, models such as Recurrent Neural Networks (RNNs) and Long Short-Term Memory (LSTM) networks began to dominate the field, enabling the generation of contextual text that far surpassed earlier attempts. However, the major breakthrough came with the introduction of Transformer models, notably the Generative Pre-trained Transformer (GPT) series developed by OpenAI.
Key Technologies
- Transformers: Introduced in the paper "Attention Is All You Need" by Vaswani et al. (2017), the Transformer architecture revolutionized NLP. By employing self-attention mechanisms, Transformers can weigh the importance of different words in a sentence, allowing them to generate coherent and contextually relevant text.
- Pre-training and Fine-tuning: Models like GPT-3 and BERT utilize a two-step process of pre-training on vast amounts of text data, followed by fine-tuning on specific tasks. This dual approach allows these models to produce high-quality text across various domains.
- Reinforcement Learning from Human Feedback (RLHF): While models can generate text based on training data, RLHF enables systems to learn from user interactions and preferences. This method helps fine-tune responses to be more aligned with human expectations and sensibilities.
Applications
The applications of text generation technologies are diverse and ever-expanding. Some notable use cases include:
- Content Creation: Businesses leverage text generation to produce articles, product descriptions, and marketing materials efficiently. This not only saves time but also helps maintain a consistent brand voice.
- Customer Service: Chatbots powered by text generation can engage with customers in real time, answering queries and providing support. These systems can handle hundreds of inquiries simultaneously, improving customer satisfaction and reducing operational costs.
- Education: Text generation is being implemented in educational tools to create personalized learning experiences. Systems can generate quizzes, summaries, and explanatory text that cater to individual learning paces.
- Creative Writing: Authors and screenwriters are using AI-generated texts for brainstorming and generating ideas. These systems can provide plot suggestions, dialogues, and narrative structures, acting as collaborative partners in the creative process.
- Translation and Summarization: Text generation models have improved the quality of machine translation and summarization services, making it easier to consume and understand vast amounts of information quickly.
Challenges
Despite its potential, text generation comes with several challenges:
- Quality and Coherence: While models like GPT-3 can produce high-quality text, they may still generate nonsensical or irrelevant content. Ensuring consistency and coherence in longer texts remains an area needing improvement.
- Ethical Concerns: The ability of AI systems to produce human-like text raises ethical questions, including the potential ChatGPT for legal document drafting misinformation, plagiarism, and misuse in creating fake news or misleading content.
- Bias and Fairness: Text generation models can inadvertently perpetuate biases present in their training data, leading to issues of fairness and representation. Addressing biases in AI-generated content is a critical area of ongoing research.
- User Trust: As the capabilities of text generation systems grow, so does the concern over the authenticity of content. Building user trust in AI-generated texts requires transparency and mechanisms to verify authenticity.
Case Study: OpenAI’s GPT-3
One of the most notable advancements in text generation is OpenAI's GPT-3, launched in 2020. With 175 billion parameters, it became the largest language model of its time, capable of producing remarkably coherent and contextually appropriate text.
Development and Technology
GPT-3 was built upon its predecessor, GPT-2, enhancing its architecture and training methodology. The model underwent extensive pre-training on diverse internet text, enabling it to understand various topics and writing styles. This vast dataset included a wide array of sources, contributing to its knowledge and flexibility.
Applications of GPT-3
- Business Applications: Companies like Copy.ai and Jasper.ai integrate GPT-3 in their platforms to offer businesses tools for generating marketing copy, blog posts, and social media content.
- Educational Tools: Websites such as Quizlet utilize GPT-3 to create personalized study materials and flashcards. The model generates prompts based on user queries, enhancing the learning experience.
- Gaming: Game developers have started to use GPT-3 for generating dialogues and narratives within games, creating more immersive and unpredictable storytelling experiences.
User Engagement and Feedback
OpenAI implemented a beta testing phase for GPT-3 to collect user feedback and refine the model. Users praised its ability to generate human-like text but also pointed out issues related to coherence over extended pieces and the occasional generation of biased content. OpenAI has since focused on implementing safety protocols and guidelines to address these concerns.
Future Prospects
The future of text generation looks promising, with several areas ripe for exploration:
- Multimodal Models: Combining text with images or audio for richer content generation is an emerging trend. Models like DALL-E and CLIP are paving the way for more integrated AI systems.
- Domain-Specific Models: Tailoring text generation models for specific industries (healthcare, law, etc.) can enhance their effectiveness and reliability in producing relevant content.
- Improved Human-AI Collaboration: As text generation models evolve, the focus will shift towards enhancing their role as collaborative tools rather than mere generators. This symbiotic relationship could revolutionize creative industries.
- Regulations and Ethical Frameworks: As AI technologies advance, the establishment of guidelines and regulations will be essential to ensure ethical practices in text generation, particularly concerning misinformation and bias.
Conclusion
Text generation has come a long way from its rule-based origins to becoming an integral part of various industries. With technologies like Transformers and models such as GPT-3, the capabilities of AI in generating human-like text have expanded dramatically. However, challenges remain, including quality issues, ethical concerns, and user trust. As the field continues to advance, the future of text generation holds immense potential for enhancing productivity, creativity, and communication across a multitude of sectors. Designing responsible and robust frameworks to guide this technology will be crucial as we navigate the next chapter of AI-driven text generation.